AI is no longer an experiment. It’s a business imperative.
From customer support automation to predictive analytics, enterprises are realising that scattered pilots or siloed tools are not enough. What’s needed is enterprise-grade AI adoption, a scalable, sustainable approach that transforms not just operations but entire business models.
Research by Everest Group and Unisys shows that companies moving beyond the “proof-of-concept” stage are seeing measurable improvements in revenue growth, cost reduction, and decision-making speed.
This blog lays out a proven framework for scaling AI at the enterprise level, one that drives measurable outcomes, improves agility, and delivers sustainable competitive advantage.
Why Scale Matters: Moving Beyond Pilots
Many organisations today have “AI on paper”, a handful of proof-of-concept projects, some RPA bots, maybe a customer-facing chatbot. But these fragmented efforts rarely deliver business-wide transformation. The issue? AI isn’t embedded; it’s being tested.
This leads to what experts call pilot purgatory, where innovation stalls due to limited scope, unclear ROI, and lack of infrastructure readiness. Despite promising use cases, these efforts often fail to deliver sustainable impact because they’re treated as side projects, not strategic priorities.
When AI is scaled, the mindset changes. Businesses move from asking “Can we automate this task?” to “How can we redesign this entire process using AI?” This shift opens the door to true transformation – faster decisions, leaner operations, and stronger customer experiences.
Isolated AI vs. Scaled AI
Factor | Isolated AI Projects | Scaled AI Adoption |
Scope | Department-level or function-specific | Cross-functional and enterprise-wide |
Data Integration | Limited to siloed sources | Unified, real-time, and interoperable |
Technology Stack | Point solutions | Cloud-native, modular, MLOps-enabled |
ROI Visibility | Hard to measure | Clear, ongoing impact on KPIs |
Sustainability | Short-term funding, experimental mindset | Long-term vision, dedicated AI investment |
Decision-Making | Manual overrides and limited automation | Augmented analytics and AI-assisted workflows |
Ultimately, scaling AI is not about technology alone, it’s about structure, leadership, and clarity of purpose. Without scale, AI remains an isolated advantage. With scale, it becomes a systemic force multiplier.
The Scalable AI Adoption Framework
Scaling AI is not a plug-and-play activity, it’s a business-wide transformation. Organisations that achieve meaningful outcomes don’t treat AI as a technology initiative. Instead, they adopt a framework grounded in strategic alignment, infrastructure readiness, culture change, and measurable impact.
Here’s a breakdown of the 7 foundational pillars for scalable AI adoption:
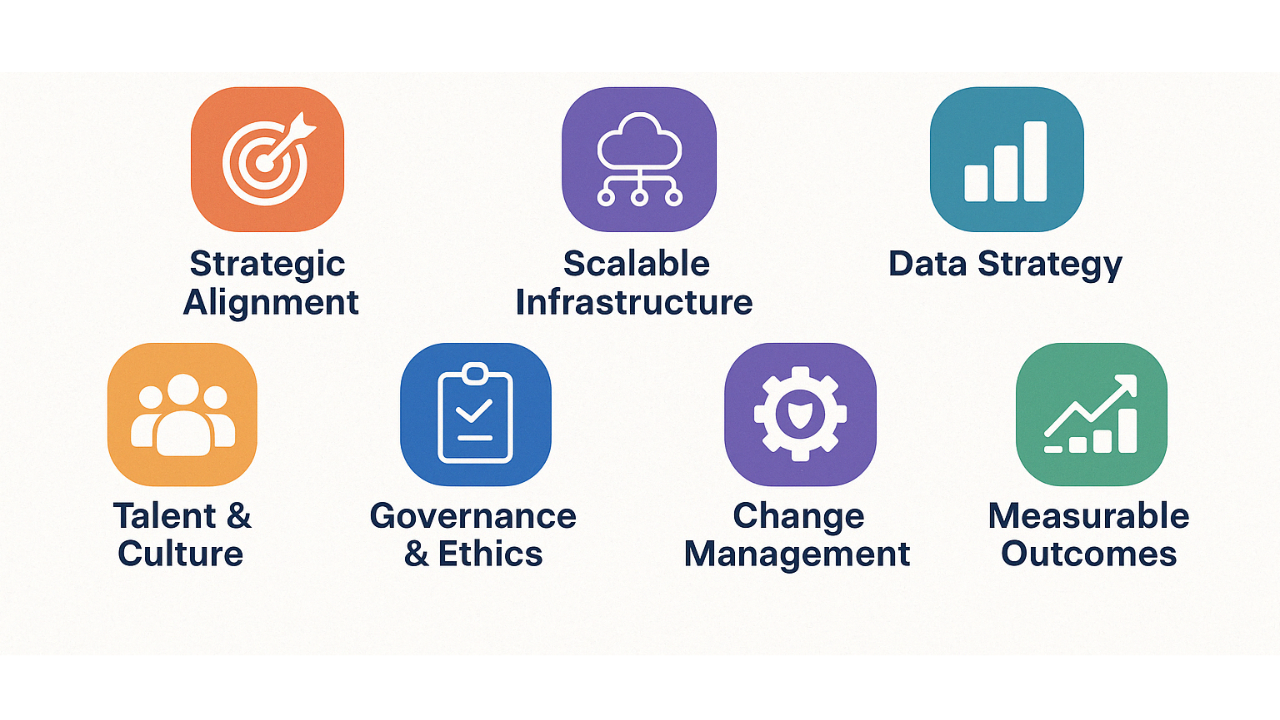
Pillar | Description |
Strategic Alignment | Align AI initiatives with business goals, customer outcomes, and KPIs |
Scalable Infrastructure | Build flexible, cloud-based platforms for model deployment and MLOps |
Data Strategy | Ensure data availability, quality, and interoperability across the organisation |
Talent & Culture | Upskill teams, promote AI literacy, and foster a culture of experimentation |
Governance & Ethics | Define responsible AI policies, compliance protocols, and risk management |
Change Management | Implement structured change strategies and cross-functional communication |
Measurable Outcomes | Track value via KPIs, efficiency metrics, and strategic impact |
Let’s Dive Deeper Into Each Pillar
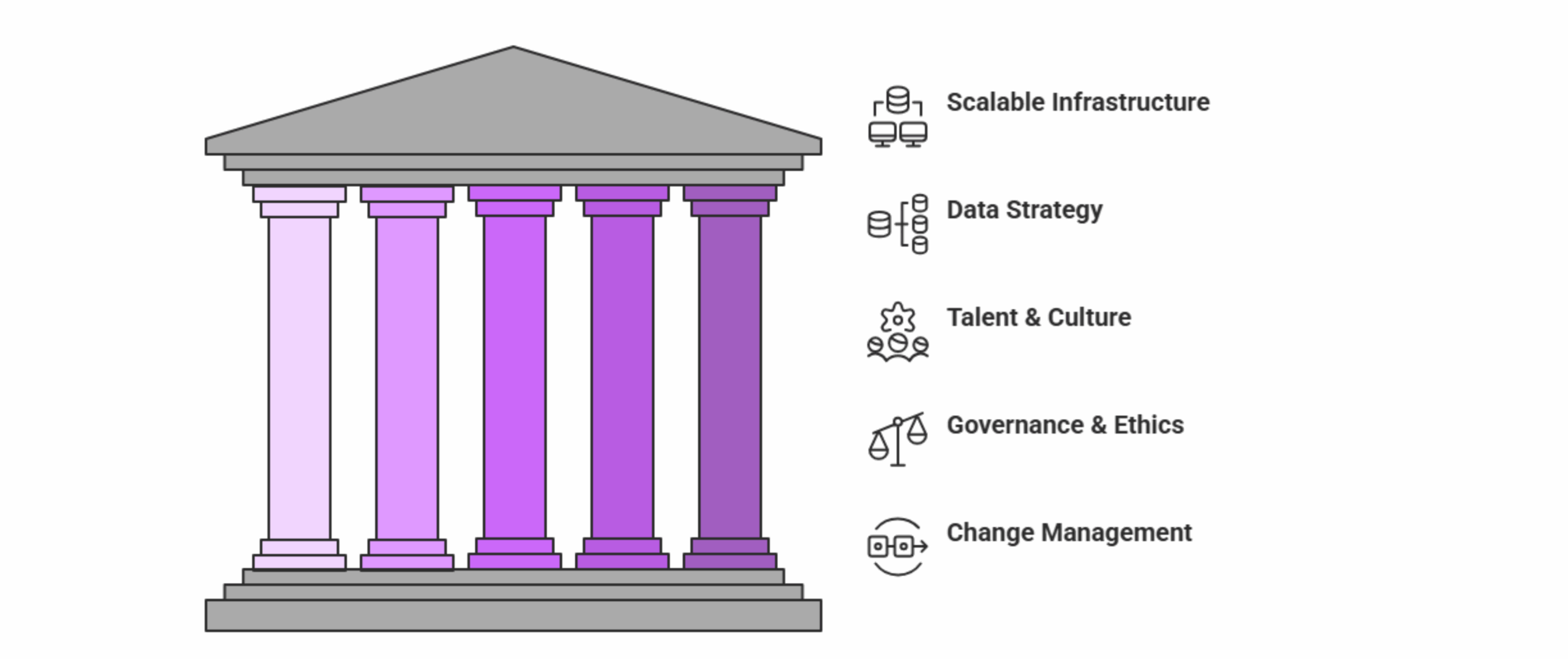
1. Strategic Alignment
AI efforts must be tied directly to business goals, whether it’s improving customer retention, accelerating product delivery, or reducing operational costs. Every AI project should answer: How does this support our mission and impact business metrics?
2. Scalable Infrastructure
Modern, flexible tech architecture is non-negotiable. That includes:
- Cloud-native platforms for compute scalability
- API-based integrations for extensibility
- MLOps practices to move models from the lab to production reliably
Without this, even the best algorithms can’t make it to production.
3. Data Strategy
AI runs on data, but not just any data. Clean, governed, real-time, and interoperable data is critical. A centralised data lake or lakehouse, real-time pipelines, and universal data standards (e.g., CDM) are the foundation for scalable AI success.
4. Talent & Culture
AI is a team sport. You need both:
- AI Specialists (e.g., data scientists, ML engineers)
- Business Translators who bridge the gap between tech and operations and beyond roles, fostering a culture of curiosity, experimentation, and cross-functional collaboration, are essential.
5. Governance & Ethics
Responsible AI isn’t optional, it’s a must. Organisations must:
- Create clear guidelines for model use
- Establish ethical review boards
- Monitor models for bias and drift. This not only builds trust but ensures compliance with emerging AI regulations.
6. Change Management
Even the best AI strategy can fail without organisational readiness. Leaders must communicate the why, offer support systems, and engage employees early. Empower “change champions” across departments to scale adoption organically.
7. Measurable Outcomes
What isn’t measured won’t improve. Define success in terms of:
- Cost reduction
- Productivity gains
- Customer experience lift: Tie each AI initiative to KPIs and ensure there’s a continuous loop of measurement, learning, and optimisation.
Multi-Phase AI Adoption Roadmap
Successfully scaling AI isn’t a single leap, it’s a structured climb. Organisations that achieve measurable impact from AI typically follow a multi-phase roadmap, ensuring they lay the groundwork before accelerating adoption.
This section outlines six critical phases to move from experimentation to enterprise-wide integration.
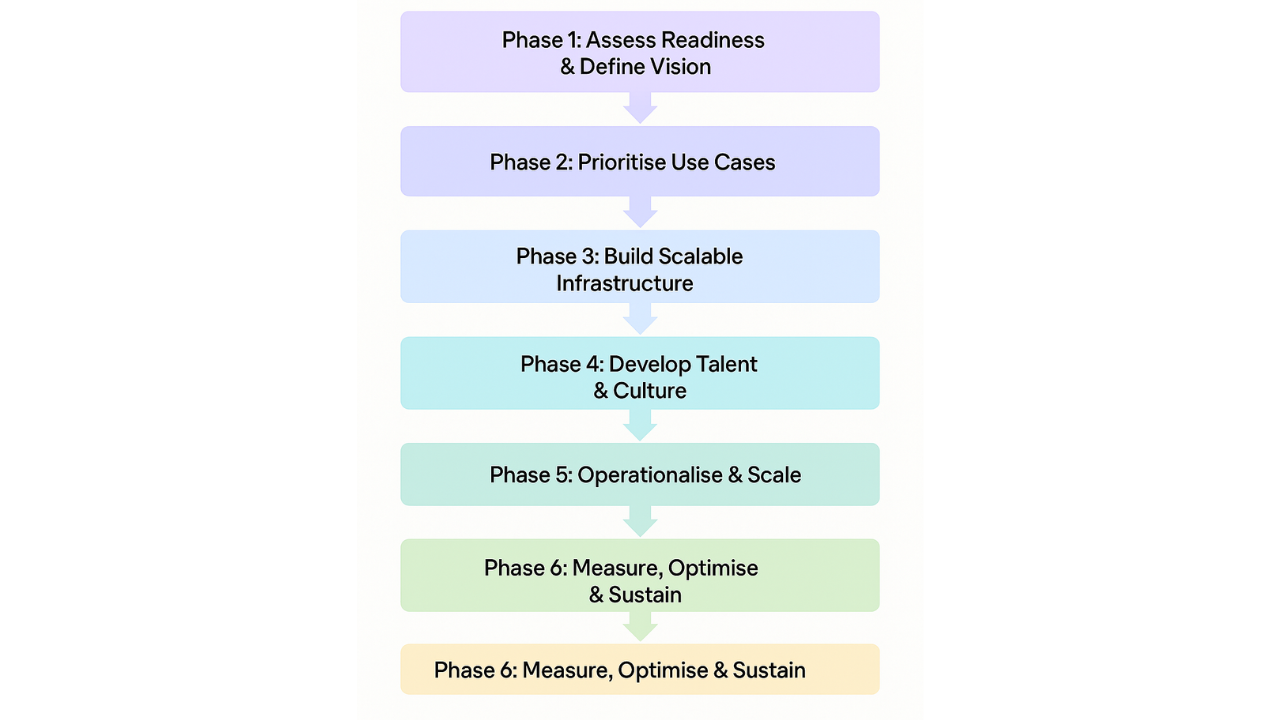
Phase 1: Assess Readiness & Define Vision
Start with a clear-eyed audit of where you are today:
- What is your AI maturity level?
- How ready is your data infrastructure?
- Do you have executive alignment?
Use these inputs to craft a vision that goes beyond tech implementation, one that ties AI to strategic goals like operational efficiency, customer experience, or new revenue streams.
Phase 2: Prioritise Use Cases
Identify and score potential AI use cases based on:
- Business value
- Feasibility
- Data availability
- Time to impact
Start small but think big. Early wins build momentum, but the end goal should be full integration into core business workflows, not just surface-level automation.
Phase 3: Build Scalable Infrastructure
Lay the foundation for AI at scale:
- Shift to cloud-native environments
- Enable elastic computing and storage
- Adopt MLOps pipelines for faster model deployment
- Integrate AI tools via secure APIs
Ensure data pipelines are reliable and can support real-time analytics.
Phase 4: Develop Talent & Culture
Train employees across three layers:
- AI Leaders – Strategy and decision-making
- Practitioners – Data scientists, ML engineers
- Business Users – AI literacy for daily workflows
Promote cross-functional collaboration and experimentation as part of your organisational DNA.
Phase 5: Operationalise & Scale
Move from pilot projects to enterprise-wide deployment:
- Integrate AI into CRMs, ERPs, and business logic
- Create playbooks for repeatable implementation
- Deploy governance protocols for risk control
Ensure transparency in how AI decisions are made and monitored.
Phase 6: Measure, Optimise & Sustain
Define KPIs tied to business goals, e.g., cost per lead, customer satisfaction, and production efficiency. Build feedback loops to:
- Continuously optimise model performance
- Sunset underperforming use cases
- Scale successful ones across departments
ROI Levers: What Scaled AI Unlocks
Scaling AI is not just about doing things faster, it’s about doing them smarter, cheaper, and with greater strategic impact. When organisations shift from isolated AI deployments to integrated systems, the business value multiplies across several dimensions:
1. Operational Efficiency
AI automates repetitive tasks, optimises workflows, and reduces bottlenecks. In manufacturing, for example, predictive maintenance powered by machine learning can reduce downtime by 20% and extend asset life by 15%.
2. Cost Optimisation
AI allows for real-time adjustments in resource allocation, be it inventory, ad budgets, or support staff. AI-enabled financial firms report up to 30% savings by automating fraud detection, compliance checks, and reporting workflows.
3. Customer Experience Enhancement
Personalisation engines powered by AI improve satisfaction and conversions. E-commerce brands that deploy AI-driven recommendation systems see an average 25% increase in average order value and a significant reduction in cart abandonment.
4. Decision Intelligence
With real-time insights, business leaders no longer have to rely on gut instinct. AI-powered dashboards enable faster and more informed decisions, improving agility in dynamic markets like logistics, retail, and energy.
ROI Table: Real-World Examples Across Industries
Industry | AI Use Case | Business Impact | Time to Value |
Retail | Product recommendations | +25% average order value | 4–6 months |
Healthcare | Diagnostic support tools | +40% faster diagnosis, improved accuracy | 6–8 months |
Finance | Fraud detection + compliance | ~$2M savings annually | 3–5 months |
Manufacturing | Predictive maintenance | -20% downtime, +15% equipment life | 6–9 months |
Logistics | Route optimisation via AI | -30% fuel cost, +18% delivery accuracy | 4–6 months |
B2B SaaS | AI-powered lead scoring | +20% sales productivity, +12% higher win rates | 5 months |
Critical Success Factors for Scaled AI
Successfully scaling AI requires more than just data and models. It demands strong foundations across leadership, culture, process integration, and partnership ecosystems. Here are the key success factors that differentiate high-performing AI organisations from the rest:
- Leadership Commitment: AI needs champions at the top. Senior leaders must not only fund AI initiatives but actively shape the strategic vision, remove barriers, and communicate its value across the organisation. Without leadership alignment, AI remains fragmented.
- End-to-End Integration: AI should be woven into the fabric of your business, not added on. This means embedding models into core processes, systems (e.g. CRMs, ERPs), and workflows where decisions are made in real time.
For example, embedding AI into supply chain tools (rather than treating them as standalone dashboards) leads to dynamic demand forecasting, not just data reporting.
- Agile Governance: Scaling AI requires both speed and control. Establish clear policies around model approval, testing, deployment, explainability, and drift monitoring. A central AI governance board can ensure accountability while enabling fast iteration.
- Cross-Functional Collaboration: Scaling doesn’t happen in silos. Business, IT, data science, operations, and compliance teams must collaborate from day one. Agile cross-functional pods often outperform traditional top-down structures when it comes to AI delivery.
- Change Management Readiness: AI transformation impacts people as much as processes. Clear communication, proactive reskilling programs, and cross-functional training help reduce resistance and build trust among stakeholders.
- Metrics-Driven Decision Making: Scaling AI requires disciplined measurement. Organisations must set clear KPIs, track performance against goals, and continuously refine based on feedback and outcomes.
- Strategic Partnerships: Most successful AI initiatives involve a blend of internal capability and external expertise – cloud vendors, AI consultancies, and research labs. Choosing the right partners can accelerate scalability and bring in valuable learnings from adjacent industries.
AI doesn’t scale on ambition alone. It requires structure, sponsorship, and sustained collaboration. The organisations that master these success factors are the ones that turn AI into a core business capability, not just a technology upgrade.
Real-World Implementation Snapshot
Case Example: AI in Supply Chain Optimisation
- A global logistics provider used AI for demand forecasting.
- Result: Reduced transportation costs by 18%, increased delivery accuracy by 22%.
- Framework in Action: Strategic alignment (efficiency), scalable data pipelines, measurable KPIs.
Case Example: AI-Powered Marketing in Telecom
- A telecom leader deployed generative AI to create hyper-personalised campaigns.
- Result: 30% increase in customer retention.
- Key Pillars Used: Talent & Culture, Scalable Infra, Measurable Outcomes.
Conclusion: Turning AI Frameworks Into Business Outcomes
AI at scale is not a technical implementation, it’s a strategic shift.
With the right framework in place, organisations can transform fragmented experiments into enterprise-wide value drivers. By aligning AI with business goals, investing in scalable infrastructure, nurturing talent, and establishing strong governance, companies don’t just deploy AI, they industrialise it.
Those who build AI as a core capability will see gains in speed, cost-efficiency, and decision-making that compound over time. The future isn’t just AI-powered. It’s framework-enabled, outcome-driven, and built to scale.
Ready to scale your AI journey? Let’s build the framework together.