In an age where businesses produce more data than ever, finding and using the correct information at the right time is becoming a competitive advantage. Yet, most organisations fail to meet this basic operational need.
According to McKinsey, employees spend nearly 20% of their workweek, equivalent to an entire workday, searching for information that already exists within the organisation. That’s not a data problem, it’s a knowledge crisis.
This breakdown stems from several compounding issues:
- Terabytes of unstructured data are stored across emails, wikis, PDFs, and tools.
- Siloed knowledge is fragmented across departments and platforms.
- Outdated search systems that rely on keyword matching, not contextual understanding.
- Loss of institutional knowledge occurs when experienced team members leave.
In short, modern organisations are rich in data but poor in usable knowledge. Traditional knowledge bases and static repositories are no longer enough.
What Are AI-Powered Knowledge Bases?
An AI-powered knowledge base is not just an upgraded FAQ system or document library, it’s a context-aware, intelligent layer that connects, understands, and retrieves enterprise knowledge across platforms and formats.
Key Features That Set Them Apart:
- Multi-source integration: Pulls content from emails, chats, CRMs, wikis, databases, and file systems.
- Contextual understanding: Goes beyond keywords to interpret intent and relationships between data points.
- Direct answers, not links: Synthesises knowledge to respond in natural language.
- Continuous learning: Improves based on user feedback and interaction patterns.
- Conversational access: Users can query the system via chat, voice, or embedded apps.
How Traditional vs AI Knowledge Bases Compare?
The evolution from static knowledge systems to intelligent, AI-powered knowledge infrastructure represents a foundational shift in how businesses manage and scale information.
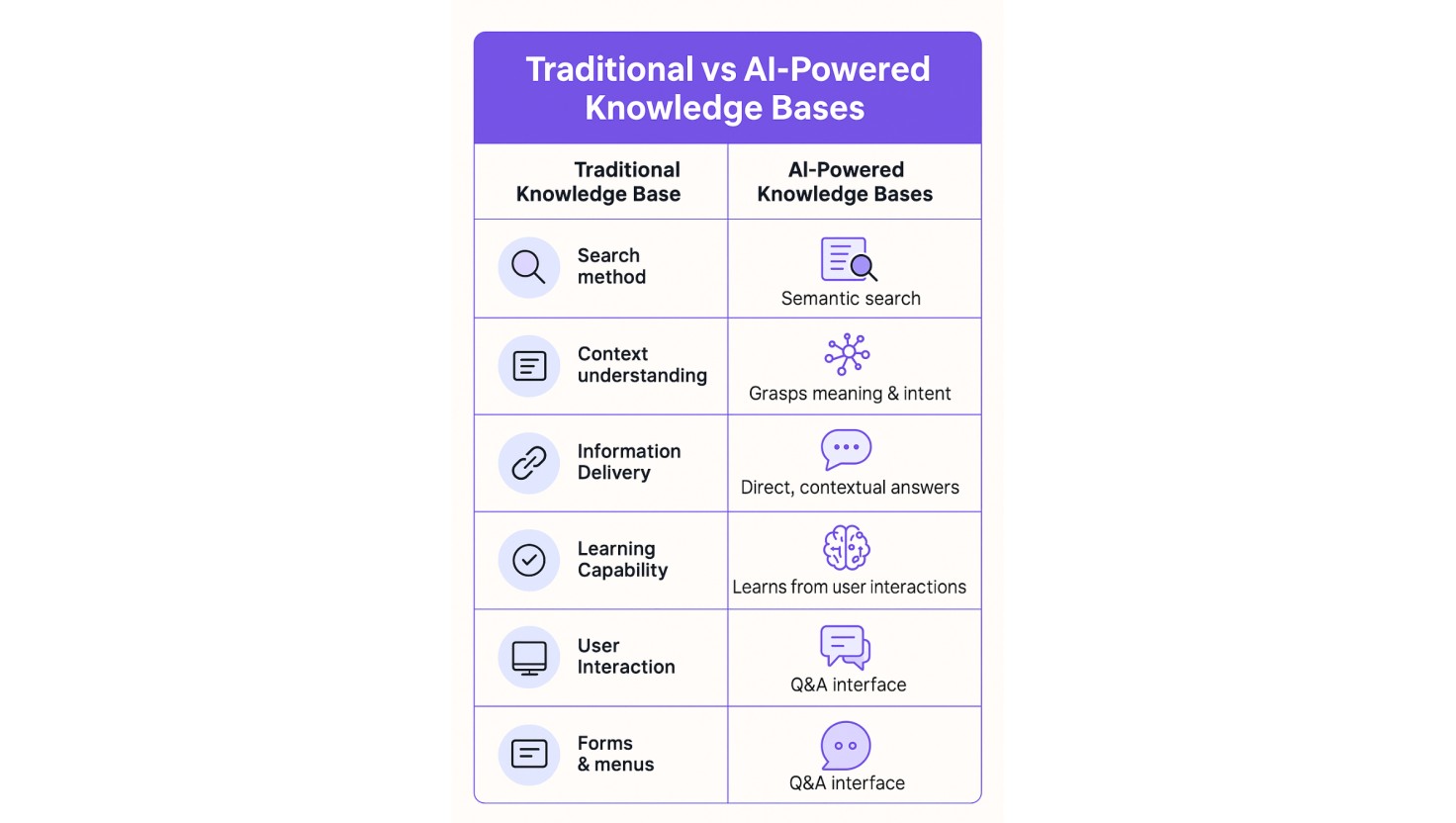
Traditional knowledge bases typically function as passive repositories, searchable collections of documents that require users to know what they’re looking for. They depend on manual tagging, keyword matching, and structured inputs, which often return broad or irrelevant results. Users are left navigating outdated files, fragmented content, and disconnected systems.
In contrast, AI-powered knowledge bases are designed to understand user intent, synthesise meaning from unstructured data, and deliver precise, contextual responses. They transform fragmented information into a unified, accessible intelligence layer that learns and improves with use.
Key dimensions of difference include:
- Search Intelligence: While traditional systems match words, AI interprets meaning using Natural Language Processing (NLP) and semantic indexing, leading to more accurate results with less user effort.
- Context Awareness: AI tools identify relationships between documents, user roles, and queries. This enables them to deliver answers relevant to the user’s task, department, or even geographic location.
- Information Output: Instead of directing users to a document, AI-powered systems generate consolidated answers drawn from multiple sources, dramatically reducing the time spent piecing together information.
- Learning Capability: Unlike traditional repositories that degrade over time, AI knowledge bases continuously refine their accuracy by learning from feedback, interaction patterns, and evolving data inputs.
- User Accessibility: AI tools are embedded directly into the platforms teams already use – Slack, Teams, Chrome, internal portals, creating seamless access without disrupting workflow.
This shift is not simply technological – it’s transformational. Organisations that replace static systems with adaptive knowledge bases don’t just reduce friction; they unlock faster decisions, lower support costs, and more scalable operations.
The Technologies Powering Intelligent Knowledge Systems
AI-powered knowledge bases are possible because of a stack of advanced technologies that collectively enable contextual understanding, accuracy, and continuous improvement.
Core Technologies Behind AI Knowledge Bases
Technology | Function |
Natural Language Processing | Interprets human queries and document content beyond simple keyword matches |
Vector Databases | Organises information by meaning, not syntax, enabling semantic search |
Retrieval-Augmented Generation (RAG) | Combines real-time document retrieval with generative AI for accurate responses |
Knowledge Graphs | Visual maps of how facts, terms, and documents relate to one another |
Conversational Memory | Maintains context across user interactions for fluid follow-up questions |
These systems don’t just retrieve — they reason. By mapping relationships, ranking sources by reliability, and understanding organisational context, AI knowledge bases deliver fewer, faster, and more accurate answers than any human searcher could.
The Business Impact of AI Knowledge Bases
AI-powered knowledge bases aren’t just technical upgrades, they deliver tangible business value that compounds across departments. From productivity gains and cost savings to faster decision-making and employee enablement, these systems help organisations unlock the full potential of their information assets.
Whether running a support-heavy operation or managing a complex product development cycle, the impact is measurable and scalable.
ROI Benefits of AI Knowledge Bases
Impact Area | Traditional Approach | With AI Knowledge Base | Estimated Value |
Employee Productivity | Time lost searching across systems | Direct answers via conversational interface | +8–10 hrs/month saved/employee |
Support Resolution | Multiple follow-ups and long wait times | Instant access to policies, procedures, and fixes | Up to 70% faster resolution |
Onboarding | Manual training, delayed productivity | Contextual answers + role-based knowledge delivery | 40% reduction in time-to-productivity |
Knowledge Retention | High dependency on senior staff | Institutional knowledge preserved in AI-readable form | Reduced turnover impact, better continuity |
Internal Ticket Volume | Employees escalate basic queries to IT/HR/helpdesk | Self-service through accurate AI responses | 30–50% drop in ticket volume |
Decision-Making | Fragmented data leads to slower or riskier decisions | Unified, real-time access to organisation-wide knowledge | Higher-quality decisions, less rework |
These benefits scale with team size. For a mid-sized organisation with 1,000 employees:
- $ 2.4 M+ annual savings can be achieved just from reclaimed employee time
- Customer service NPS and CSAT scores improve due to consistent and fast responses.
- Cross-functional efficiency improves when teams no longer operate with outdated or conflicting information.
How Different Teams Leverage AI Knowledge Bases?
AI-powered knowledge bases aren’t one-size-fits-all; they adapt to each department’s needs. Whether they accelerate customer service, enable sales reps, or help engineers resolve technical issues faster, the impact is both operational and strategic.
Below is a breakdown of how different teams benefit from intelligent knowledge systems:
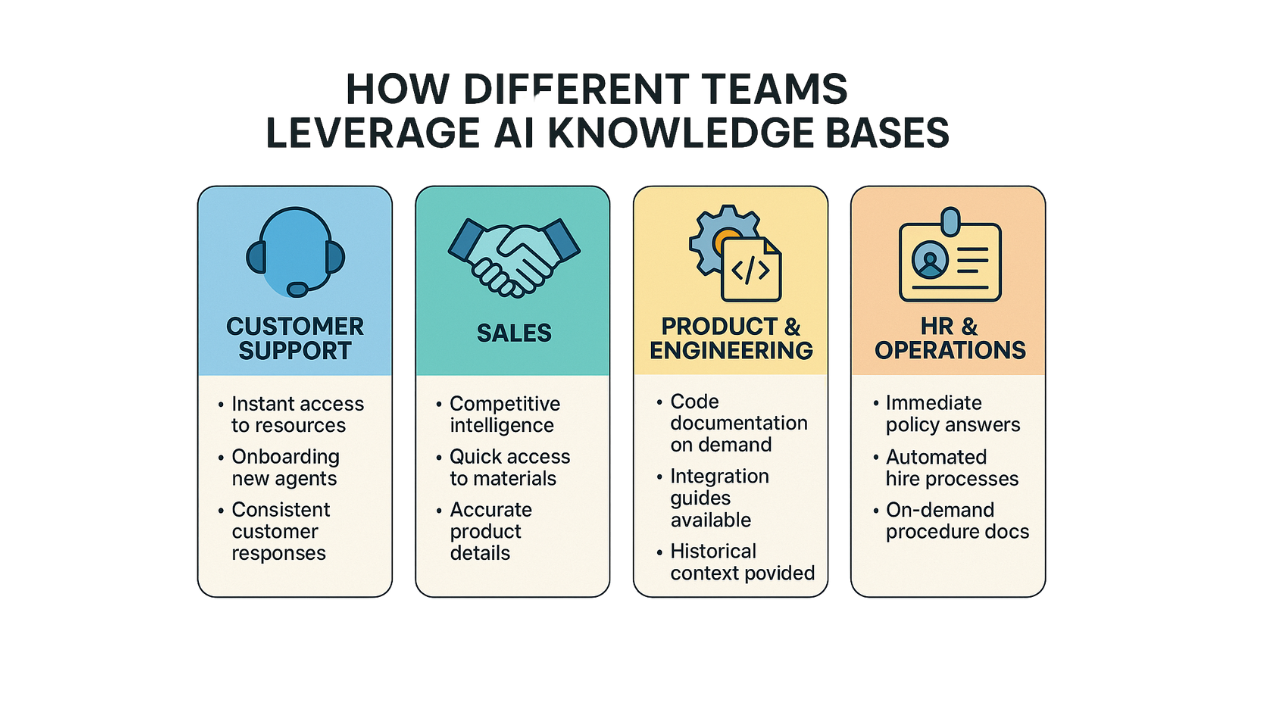
Customer Support: Instant Resolutions, Consistent Messaging
- Immediate access to product manuals, refund policies, and troubleshooting guides.
- AI suggests real-time answers across chat, email, and voice channels.
- Consistent responses across Tier 1 and Tier 2 queries.
- Training new agents takes days, not weeks.
- Stat to cite: Up to 70% faster ticket resolutions.
Sales: Informed Reps, Shorter Sales Cycles
- Real-time access to competitor insights, pricing policies, and case studies.
- Reps receive in-call knowledge suggestions and playbook prompts.
- Reduces ramp-up time for new hires by 40–50%.
- Avoids delays caused by dependency on pre-sales or solution engineering.
Product & Engineering: Faster Dev Cycles, Fewer Bottlenecks
- Instant access to API docs, architecture diagrams, and historical feature decisions.
- Surfaces past bug fixes, version logs, and integration lessons.
- Reduces repetitive technical queries within engineering teams.
- Helps new devs get up to speed on legacy systems or technical debt areas.
HR & Operations: Self-Service, Policy Consistency
- Common questions around leave policy, benefits, and onboarding are handled instantly.
- Reduces reliance on the HR team for repetitive queries.
- Ensures uniform understanding of procedures across all teams.
- Helps standardise knowledge in fast-growing or distributed teams.
These use cases illustrate how an AI knowledge base becomes more than a static FAQ – a live, always-on knowledge partner embedded across the business.
Implementation Strategy: Deploying AI Knowledge Bases Effectively
Successful adoption of AI knowledge systems isn’t just about choosing the right technology, it’s about aligning it with real workflows, high-impact problems, and measurable outcomes.
Here’s a structured approach to deploying an AI-powered knowledge base across your organisation:
1. Start with a Focused Use Case
Begin where the pain is most apparent – teams that:
- Handle repetitive, high-volume queries (e.g., support or HR).
- Rely heavily on tribal knowledge.
- Have measurable KPIs (e.g. resolution time, ticket volume).
Example: A customer support team dealing with repetitive refund policy questions is a perfect pilot group for AI-powered knowledge delivery.
2. Connect Your Knowledge Sources
Identify where your knowledge lives:
- PDFs, Google Docs, Notion pages, SharePoint, Slack threads, CRM systems Use connectors to bring these into one unified index. Tools like Lleverage.ai, ClickUp AI, and Glean support this seamlessly.
Ensure data remains synchronised and updates flow automatically – a stale knowledge base is worse than none.
3. Configure, Train, and Test
- Based on your use case and data structure, choose the right LLM (e.g., GPT-4, Claude).
- Configure prompt behaviour: tone, brevity, level of detail.
- Add fallback messages for sensitive or unsupported queries.
- Test extensively with real user queries across departments.
Pro tip: Use RAG (Retrieval-Augmented Generation) to ensure answers are grounded in your internal content and not hallucinated by the model.
4. Roll Out Incrementally
- Launch with a small group of power users or a single team.
- Monitor usage, response accuracy, and time-to-resolution metrics.
- Collect feedback on gaps, false positives, and system speed.
- Refine continuously before scaling across functions.
5. Measure What Matters
Don’t just track usage – track business value:
- Time saved per employee
- Support ticket reduction.
- Onboarding ramp-up time.
- Number of repeated queries deflected.
- User satisfaction and response helpfulness ratings.
By aligning deployment with real team needs, knowledge bases become more than just a tech upgrade – they become a workflow transformation tool.
Overcoming Common Implementation Barriers
Despite the rise of AI-powered knowledge bases, some organisations still hesitate due to legacy concerns. Here are the top barriers – and how modern platforms solve them.
1. Data Privacy and Security
Enterprises worry about exposing sensitive information. Today’s AI platforms offer:
- Zero data retention
- Private cloud or on-prem deployment
- Role-based access and encryption
- Compliance with GDPR, SOC 2, and more
These features ensure complete control over what’s indexed and who can access it.
2. Fragmented or Unstructured Data
Knowledge is often scattered across emails, PDFs, and chat tools. AI knowledge systems handle this by:
- Extracting answers from messy content.
- Mapping relationships without manual tags.
- Identifying outdated or low-confidence data.
No cleanup required – systems improve through usage and feedback.
3. User Adoption Challenges
Traditional tools often disrupt workflows. AI systems now integrate directly into:
- Slack, Teams, and CRMs.
- Help desks and browser extensions.
With conversational interfaces and real-time support, adoption becomes seamless and organic.
What’s Next: Future Trends in AI Knowledge Systems
As AI knowledge systems mature, their capabilities evolve beyond search and retrieval. Here’s what’s on the horizon for 2025 and beyond:
- Multimodal Understanding
Future systems will process not just text, but diagrams, screenshots, and videos, making knowledge more visual and accessible.
- Proactive Knowledge Delivery
AI will begin surfacing relevant insights before users ask, based on real-time context, intent signals, and behaviour patterns.
- Advanced Visualisation
Knowledge graphs will become more interactive, allowing teams to explore visually the connections between policies, decisions, and projects.
- Personalised Knowledge Experiences
Information will be tailored to the user’s role, seniority, and past interactions, streamlining relevance and reducing noise.
- Action-Oriented Agents
The next generation will go beyond answering questions by triggering workflows, filling forms, and assisting with task execution directly within the knowledge system.
Turning Information Into Scalable Intelligence
AI-powered knowledge bases are not just tools for organising documents – they are platforms for scaling expertise, accelerating decisions, and reducing operational friction.
In a world where speed, consistency, and clarity define competitive advantage, businesses implementing intelligent knowledge systems will move faster, retain more institutional knowledge, and empower employees with instant access to what they need.
The future of work is not just about working harder – it’s about working smarter with AI at the core of your knowledge strategy.
Ready to turn your knowledge into a growth engine?
Explore how NoCrew’s AI-powered solutions can help your teams scale smarter. Get in touch with us.