AI in marketing is not a single capability, it’s a spectrum. On one end lies task automation: structured, rules-based systems designed to reduce manual effort. On the other hand is machine learning: dynamic models that learn from data and adapt in real time.
Confusing the two can lead to wasted investments, misaligned expectations, or underutilised tools. For marketers navigating AI adoption, understanding this distinction is essential.
In this blog, we’ll break down the key differences, when to use each, and how the right blend can drive measurable results across your marketing funnel.
What Is Task Automation in Marketing?
Task automation refers to the use of pre-defined rules and workflows to execute repetitive marketing tasks without manual intervention. It’s often the first step in AI adoption for marketing teams, offering time savings and operational efficiency.
These systems are designed around “if-this-then-that” logic. For instance, if a user downloads an eBook, they automatically receive a follow-up email three days later. No intelligence is involved, just structured execution.
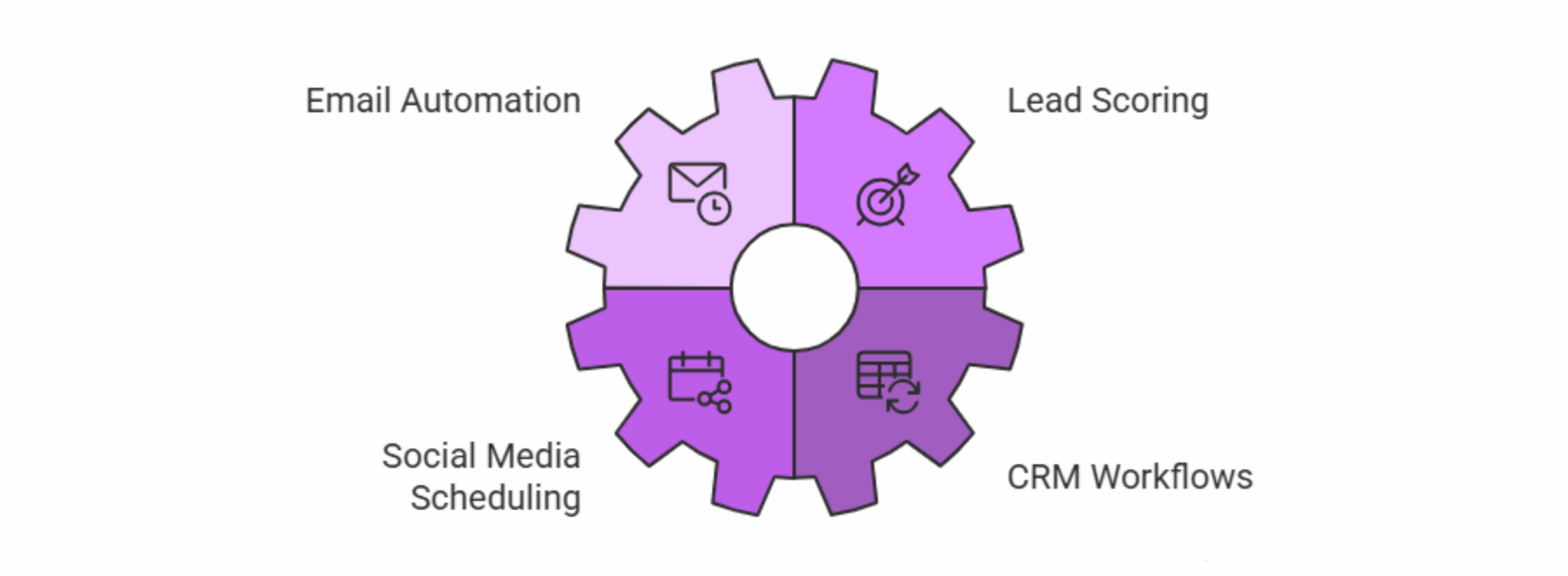
Common Use Cases:
- Email automation: Welcome series, cart abandonment emails, re-engagement campaigns
- Lead scoring: Rule-based assignment of lead value based on actions or firmographics
- Social media scheduling: Publishing content at optimal times
- CRM workflows: Assigning leads, triggering notifications, updating records
Task automation tools, like HubSpot Workflows, Mailchimp Automations, and Hootsuite, help teams scale execution without scaling headcount.
Task Automation Use Cases vs. Business Impact
Use Case | How It Works | Impact |
Welcome Email Series | Triggered when a user signs up | Boosts open rates, quick onboarding |
Lead Routing | Based on geographic or demographic rules | Faster sales response times |
Social Post Scheduler | Pre-set calendar distribution | Improves content consistency |
Loyalty Reminders | Sent after X days of inactivity | Increases repeat visits or sales |
While automation is powerful, it’s limited by logic; it cannot learn, adapt, or optimise based on changing user behaviour. For that, machine learning takes the lead.
What Is Machine Learning in Marketing?
Machine learning (ML) in marketing refers to systems that go beyond pre-set rules and instead learn from data to make predictions or decisions. Unlike automation, which follows static instructions, ML models continuously adapt based on user behaviour, campaign results, or new input signals.
At its core, ML is about pattern recognition at scale. By analysing historical and real-time data, these models uncover trends, predict outcomes, and personalise experiences automatically.
Key ML Use Cases in Marketing
- Predictive Lead Scoring: Instead of assigning points based on fixed rules, ML models learn which behaviours correlate with conversion and score leads accordingly.
- Customer Churn Prediction: Analysing behaviour signals to flag users at risk of leaving, before they actually do.
- Dynamic Personalisation: Recommending products, content, or offers based on a customer’s unique behaviour profile.
- Audience Segmentation: Clustering users into behavioural segments (not just demographic ones) for targeted messaging.
How It Works (in practice)
An ML system typically:
- Ingests raw data (CRM, website activity, campaign performance)
- Finds hidden patterns (e.g., what actions predict a conversion)
- Train on these patterns over time
- Applies its learnings to score, segment, or suggest outcomes
This is what powers tools like Salesforce Einstein, Adobe Sensei, or Google’s Performance Max campaigns. Instead of asking a human to define every rule, ML systems generate their own rules from experience.
Machine learning delivers contextual, real-time intelligence. It’s what lets top marketing teams shift from campaign planning to continuous optimisation, driven by actual audience behaviour.
Key Differences Between Task Automation & Machine Learning
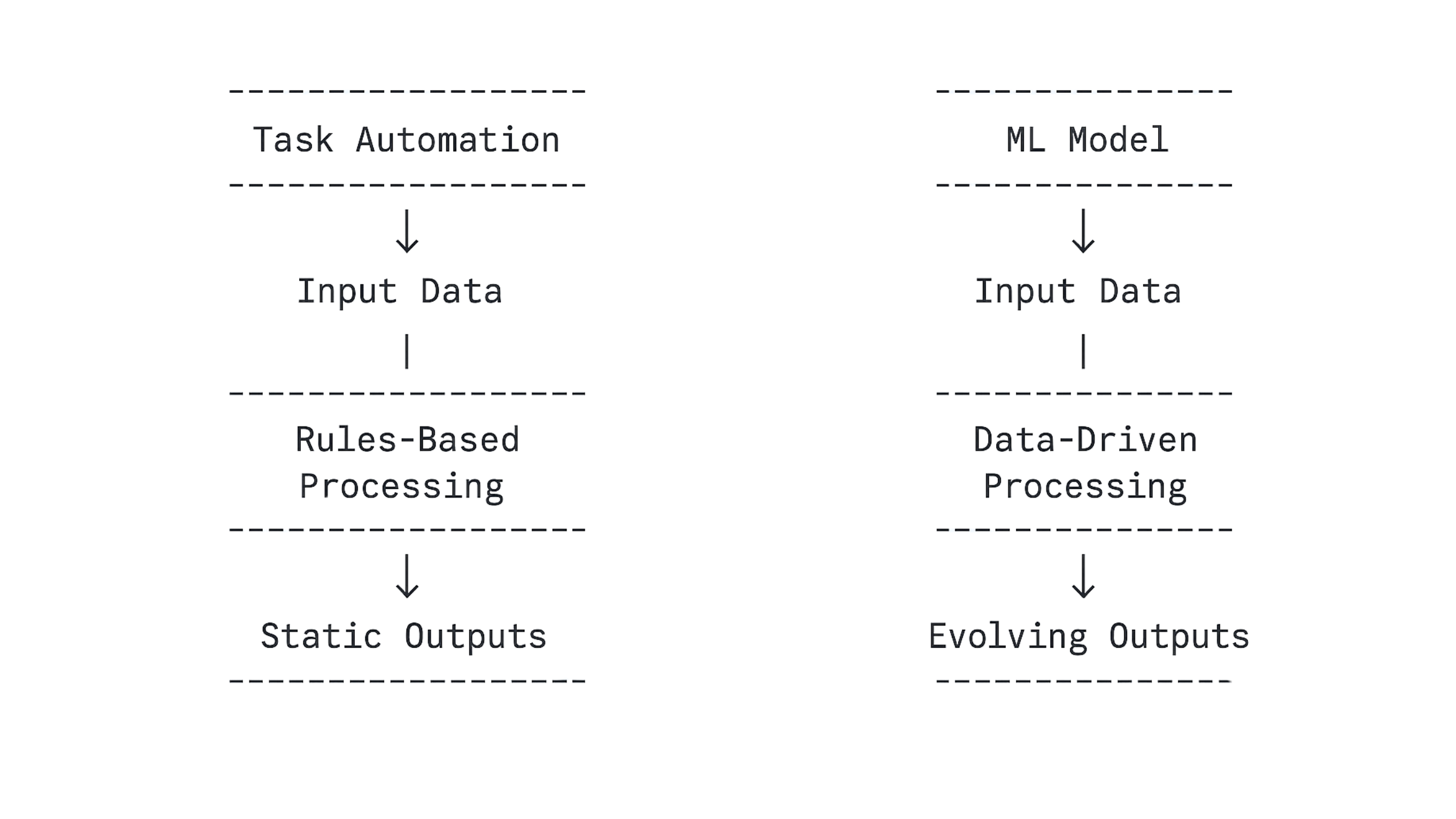
While both task automation and machine learning are used to improve marketing operations, their core mechanics and potential are fundamentally different. Here’s a breakdown of where they diverge:
1. Learning Ability
- Automation follows a set of predefined instructions. It doesn’t learn from data—it just executes rules consistently.
- Machine learning, on the other hand, improves over time by finding patterns in large datasets and adjusting outputs accordingly.
2. Adaptability
- Task automation is rigid. If customer behaviour changes, the rules must be manually updated.
- ML systems dynamically adjust based on user interactions, making them more suited to fast-changing environments like social media, paid ads, or e-commerce.
3. Personalisation Depth
- Automation can personalise at a basic level (e.g., “First Name” in emails).
- ML enables hyper-personalisation, such as predicting what content or offer will convert a specific user based on browsing and purchase history.
4. Complexity & Cost
- Automation is easier to implement and usually part of most marketing platforms.
- ML requires clean data, model training, and often third-party tools or data scientists—making it more resource-intensive but highly scalable once established.
5. Business Use Case
- Use automation for structured, repeatable tasks.
- Use ML when you need to learn from data or adapt to new patterns automatically.
Task Automation vs. Machine Learning in Marketing
Category | Task Automation | Machine Learning |
Learning | None – static rules | Learns from data continuously |
Adaptability | Manual updates required | Dynamic, auto-adjusting outputs |
Personalisation | Basic, rule-based | Hyper-personalised predictions |
Setup Complexity | Low | Moderate to high |
Use Cases | Email workflows, lead routing | Predictive scoring, churn detection, dynamic offers |
Tools | HubSpot, Mailchimp, Zapier | Salesforce Einstein, Adobe Sensei, custom LLMs |
Which One Should You Use? A Decision Framework
Not every marketing challenge requires machine learning, and not every routine can be handled with simple automation. Choosing the right approach depends on your business goals, data maturity, and the level of personalisation or insight required.
Let’s break it down:
Use Task Automation When:
- You have a clearly defined process.
- Rules rarely change and can be easily codified.
- The task is repetitive and doesn’t require learning or adaptation.
- You need to save time and reduce manual workload.
Examples:
- Welcome emails after sign-up
- Lead routing based on geography
- Auto-scheduling content on social media
Automation works best when your logic is predictable, and your objective is operational efficiency.
Use Machine Learning When:
- You have large volumes of behavioural or transactional data.
- The user journey is complex or varies widely.
- You want to optimise or personalise in real-time.
- The output depends on ongoing pattern discovery, not static rules.
Examples:
- Predicting customer churn
- Recommending next-best offers or products
- Forecasting campaign performance or conversion likelihood
ML shines when your marketing efforts demand insight, agility, and prediction.
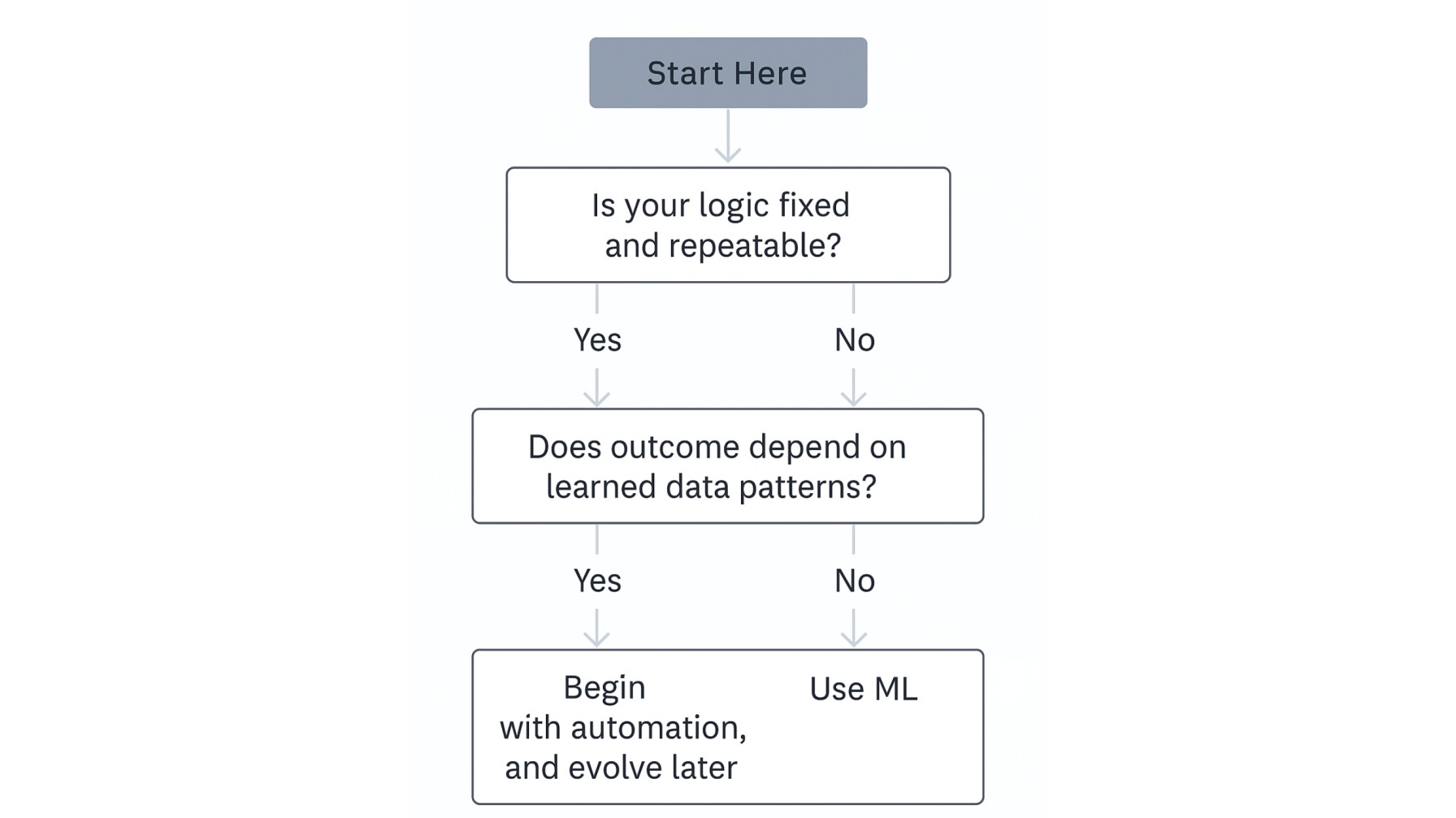
Hybrid Use Cases: The Best of Both
In many modern marketing stacks, automation and ML work together:
- A machine learning model predicts churn → automation sends a targeted re-engagement email.
- ML clusters customers by lifetime value → automation pushes tailored offers via SMS or WhatsApp.
The smartest teams integrate both layers; ML powers the intelligence, and automation powers the action.
Examples of Use Cases
Theoretical differences are useful, but what really drives the point home is seeing how brands use task automation and machine learning in action. Below are a few real-world examples across industries, showcasing where each approach thrives and when they’re best used together.
Retail Brand: Automation + ML for Personalisation at Scale
A leading fashion e-commerce platform uses automation to trigger order confirmations, return reminders, and seasonal campaigns. But to drive personalisation, it leverages ML-powered recommendation engines based on browsing history and past purchases.
Impact:
- +23% increase in average order value
- -18% cart abandonment rate
B2B SaaS: Lead Qualification with Machine Learning
A SaaS company offering productivity tools initially used static lead scoring based on firmographics (company size, industry). After switching to ML-based scoring, their system now ranks leads based on behaviour (demo engagement, email interaction, product usage).
Impact:
- 30% higher MQL-to-SQL conversion rate
- 20% increase in sales rep efficiency
Fintech Startup: Automation for Compliance, ML for Risk Prediction
A digital lending company automates document verification, KYC workflows, and customer onboarding using task automation tools. Meanwhile, it applies ML models to assess credit risk by analysing transaction history and income patterns.
Impact:
- Reduced onboarding time by 40%
- Flagged high-risk profiles 3x more accurately
Use Case Highlights
Industry | Automation Used For | ML Used For | Result |
Retail | Email campaigns, reorders | Product recommendations | +23% AOV, ↓ cart abandonment |
SaaS | Welcome flows, CRM updates | Predictive lead scoring | +30% MQL quality |
Fintech | KYC, workflow triggers | Risk profiling, fraud detection | Faster onboarding, ↑ accuracy |
Conclusion: A Balanced Approach to AI in Marketing
Task automation and machine learning are not rivals, they’re complementary tools in a modern marketer’s arsenal. Automation drives efficiency through structured, rule-based workflows. Machine learning adds depth by uncovering insights and adapting in real-time.
The smartest teams don’t choose one over the other, they deploy both strategically, based on business goals, data maturity, and the complexity of customer journeys.
By understanding their differences, you can maximise ROI, reduce manual effort, and unlock intelligent, scalable marketing systems that evolve with your audience.
Looking to evolve your marketing with AI? Start with the right foundation and scale with confidence. Get in touch with us.